Ready. Fire. Aim.
Scaling your drone program before defining 3d data requirements.
READY. FIRE. AIM.
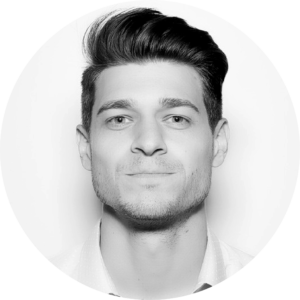
Written by
Josh Meler
Chief Marketing Officer
Of all the use cases I’ve seen, drones in Telecom are one of the most obvious. Tower sites have always been difficult to inspect, and even more challenging to quantify. The drone industry has courted Telecom for nearly a decade, and tower companies are now beginning to hire out partners to collect data across thousands of sites. But before these companies ramp up drone operations, they should define exactly how the data will be used and who will benefit from it.
Most telecom companies don’t know what a 3d reality model can do for them, and too many partners are over-promising on capabilities and under-delivering on results. The fact is analytical capabilities are a derivative of the 3d reality model that a drone collects. And until the recent emergence of Engineering Class drone data, tower companies haven’t been ready to scale drone programs because drone sensors had yet to achieve a fidelity of 3d information that is meaningful across the organization.

The current, broken model.
The current model for scale is to use a hobby-class drone. This makes sense at a high level. These off-the-shelf UAVs are affordable and widely available. If you crash one into a tower, it’s easy to buy another. Limited specialized training is required, and anyone can purchase one. The idea is you can push a button and let it go – autonomously.
This sounds great in theory, but in practice it doesn’t work in Telecom. Tower structures are dissimilar, complex, and capturing full coverage of the structure is challenging. Furthermore, the camera sensor on these drones is designed to be extremely lightweight, small, and affordable, so they appeal to the hobbyist budget too. The resulting tower data collected using these drones is spotty and imprecise. While there are certainly good 3d examples where tower data was collected in ideal conditions when wind, heat, cloud cover, and sunshine weren’t an issue – the mathematics of photogrammetry limit what is possible with these drones. And rather than modify the camera to better capture tower information, drone manufacturers and flight software companies ask operators to fly closer to structures and trust onboard sense-and-avoid systems to identify wires, cabling, and other hazards.
With all this, you still only get “inspection-class”, centimeter-accurate 3d data. Typically, there are isolated areas where accuracy might be sufficient, but data accuracy and coverage deteriorate as you move down the 3d model. You can run this data through Digital Twin software for tower analysis, but AI can’t extract dimensional measurements that are required to calculate loading and capacity. This leaves you with a high margin of error and a low-confidence result.
Site visits and legacy data are still a problem.
The problem with the current model is that you’re going to have to return to the site again to verify observations and perform millimeter-accurate hand measurements. So, Inspection class drone data doesn’t necessarily save a truck roll, it just delays it.
To account for a deficient in coverage and accuracy, the hope is to rely on notoriously poor legacy information to fill data gaps and make “best guesses”. Again, in theory there is a tower database that has complete, accurate and up-to-date information about the site. This can be used to cross-validate the lower-accuracy drone data results to feed structural information into a digital twin platform. However, in reality, poor legacy information is a shared problem in the telecom industry. Many tower sites have traded hands previously, and it’s not uncommon to find additional equipment on towers that was added erroneously or never removed. Measurements and photos are collected manually by in-house and third-party workers, then stored on notepads or personal laptops. And towers themselves undergo planned and unplanned changes over time, making the exact state of any given site difficult to predict.
If drone data doesn’t reset legacy information and provide a new baseline for as-built truth, then what is it solving for?
Scaling before defining 3d data requirements.
Why would you want to scale a drone solution that satisfies a small portion of your overall tower lifecycle data requirements, and still requires a truck roll to collect more accurate measurements by hand? The costly part of drone data collection is manpower and time. If you’re going to invest in mobilizing a workforce to capture tower data, why not collect a fidelity that’s reusable and serves new use cases that extend beyond simply field inspections?
I think we were so excited by the prospect of drones replacing climbers, that we charged right past important questions about data quality and coverage, and immediately started to talk about how to scale a telecom solution. I know drone companies were eager to have that conversation. Hence, today’s solutions largely address field operations, but neglect many other crucial lifecycle uses.
The right way to scale.
Before investing in a costly effort to collect 3d tower data from drones, first start with a list of all the possible uses for that data. Outline all the reasons towercos, carriers, turf-vendors, engineers and other field teams visit a site, and then take a close look at the data they collect and the tasks that result from that data. Then investigate what’s possible with the different classes of 3d data available, paying close attention to measurability and coverage. You’ll quickly discover that most downstream tasks are structural in nature and rely on millimeter-accurate dimensions. You’ll also discover that while most digital twin platforms can provide rich insights about tower sites, your level of confidence will only be as high as the quality of 3d data that the analysis is based on.
It’s only after you define what class of 3d data you require from drones that you can begin exploring how to mobilize drone operators to collect portfolio data.
Conclusion.
It will be game-changing when drones are commonplace on tower sites, and tower companies have a full and up-to-date library of digital twins to match their portfolios. But the first step to integrating drone data into the workstream isn’t rushing to collect tower data across as many sites as possible, and only then exploring the limitations of the data you’ve collected. This “ready, fire, aim” approach will yield data that offers a short shelf-life and requires additional site visits to verify.
On the other hand, an emerging new category of Engineering Class drone data promises to provide teams with the accuracy and coverage necessary to justify a scaled collection.
This has been the guiding principle at Visual Intelligence. Our team has built towercos from scratch, and fully understands that only Engineering Class drone data will reset legacy information and address lifecycle requirements. We spent years developing a first-of-its-kind dual-camera drone sensor that collects millimeter-accurate 3d data across 99% of a tower structure’s surface. And over the past few years, we’ve addressed the challenges of scale head on by automating the tower collection process, miniaturizing our sensor to be compatible with more aircrafts, developing a portal to manage operators and data, designing more efficient ways to process high volumes of 3d data, and by exploring digital twin partnerships that make full use of Engineering Class 3d data.